When moving analytical projects into production, they should help create value and analytical performance should be high. Data is a critical driver for creating this value. Without new data, dashboards go out of date, and models either make poor predictions or no predictions are made at all. This causes end users to lose trust. Therefore, there needs to be an area that will allow production data to reach from point A to point B when we need it - this is called DataOps.
DataOps (short for data processing and a term not to be confused with DevOps) is a discipline, set of processes and technologies that allow organizations to bring and maintain data into production, usually for their analytics projects. It can include analytical projects, production reports and dashboards to make predictions, or artificial intelligence and machine learning. Regardless of use case, analytics helps organizations make more informed decisions and deliver more engaging and valuable customer experiences. For any type of analytical project, the quality and timeliness of data is critical to production success.
DataOps Technology
In terms of technology, DataOps automates data distribution for production analytics. Visual or code-based data pipelines include a step-by-step process for linking, merging, and converting data into a functional form. DataOps transforms steps into automated production sequential layouts that run over and over again. DataOps automation requires systematic controls on metrics to look for variations in data and pipeline operation issues.
DataOps technology relies on the use of processes and proven, ideally pre-packaged functions and automation. Using a built-in data processing line framework reduces the work of developing and automating process lines and minimizes downtime due to production issues, creating more repeatable and reliable results.
DataOps with Dataiku
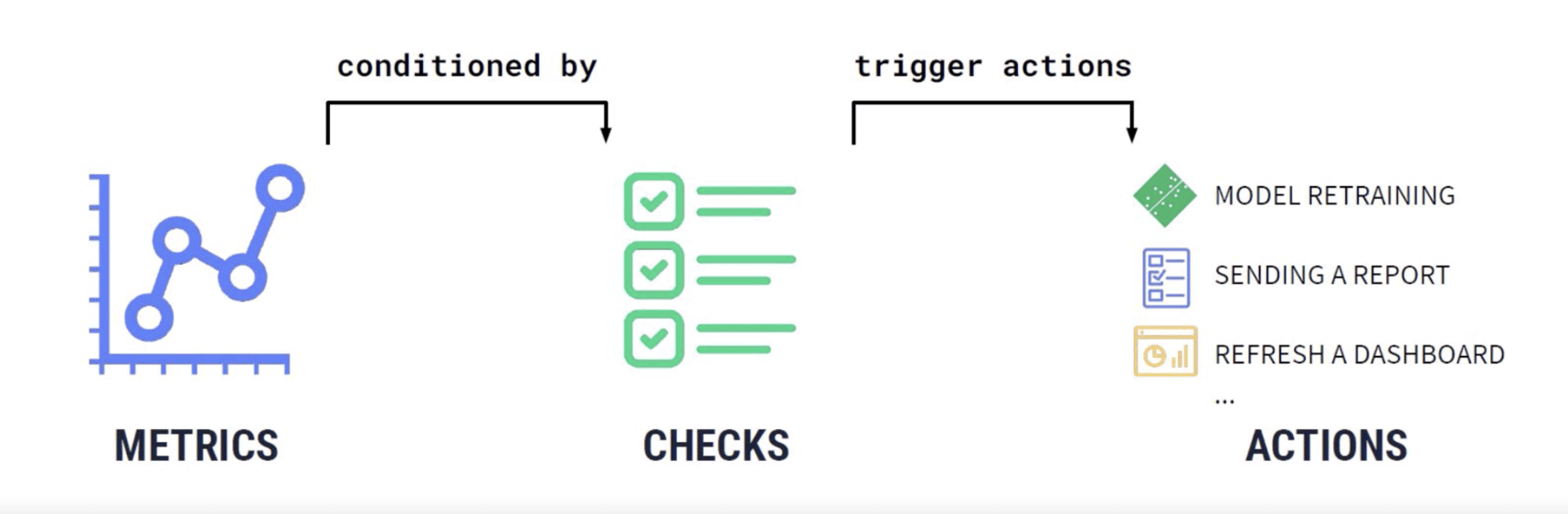
When projects move from design to production, Dataiku allows teams to quickly map production data, test projects in production environments, adjust sequential layout metrics and data quality controls, and trigger sequential layouts that will work according to a schedule or conditions.
You can send us an email about DataIku's automated solutions for DataOps and the data science & artificial intelligence processes we offer.
İlginizi Çekebilecek Diğer İçeriklerimiz
Her iki model türü de doğal dil işleme (NLP), bilgisayarla görme ve diğer alanlarda güçlü uygulamalara olanak tanır. Ancak, bu modellerin yapılarına, uygulamalarına ve avantajlarına baktığımızda belirgin farklar var.
At the Google Cloud Next '25, many innovations were announced in the field of artificial intelligence and cloud that will closely interest developers and decision-makers. In this article, we compile and summarize the main topics introduced at the event.