At the Google Cloud Next ‘25, many innovations were announced in the field of artificial intelligence and cloud that will be of great interest to developers and decision makers. In this article, we compile and summarise the main topics introduced at the event. From next-generation Gemini models to developer tools, from infrastructure improvements to multi-agent ecosystem, we will cover the prominent announcements in a short and understandable way.
New Gemini Models
- Gemini 2.5 Pro: Introduced as Google's most advanced AI model, Gemini 2.5 Pro is capable of reasoning before responding as a ‘thinking’ model. Offering up to 1 million tokens of context, it is optimised for analysing complex documents, understanding extensive code bases and performing tasks that require high precision. It promises superior performance, especially in demanding tasks such as writing/correcting detailed code or extracting critical information from medical documents.
- Gemini 2.5 Flash: The ‘ working’ model of the Gemini family, the 2.5 Flash has been optimized to offer low latency and cost advantages. Aiming to provide fast responses in daily use scenarios such as real-time summarisation or high-volume customer service interactions, the model can automatically adjust the ‘think time’ according to the complexity of the query and thus produce instant results for simple requests. Developers can control this time and depth of processing on demand in the Flash model to strike a balance between speed, accuracy and cost that suits their needs. This flexibility makes powerful AI more accessible and affordable for everyday use.
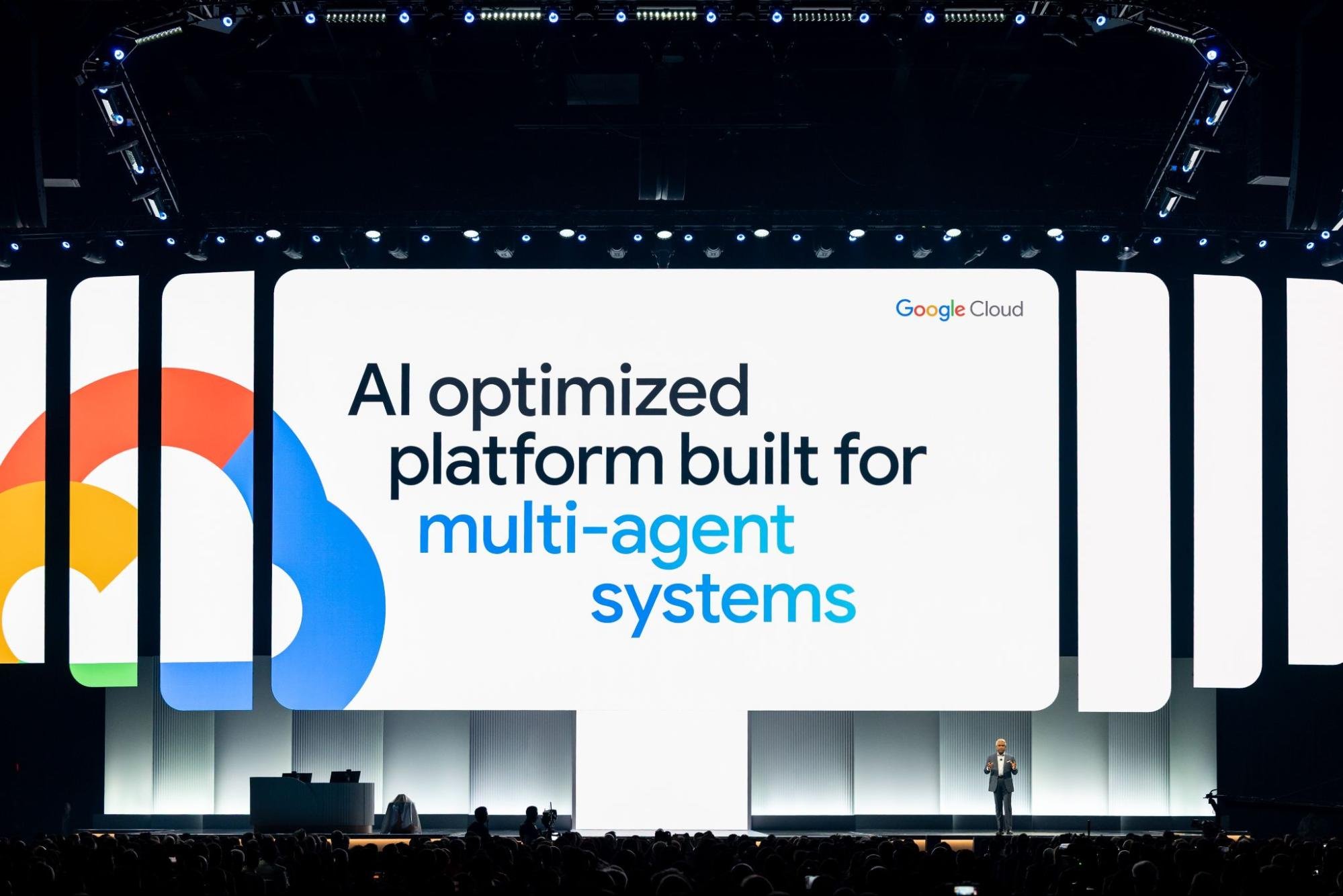
Generative AI Models (Imagen 3, Chirp 3, Lyria, Veo 2)
- Imagen 3: A new version of Imagen 3, Google's highest quality text-to-visual model, has been announced. This update includes the ability to complete missing or distorted parts of images (inpainting) and naturally remove unwanted objects. Thanks to the improvements in object removal and image editing quality, users will be able to produce much more consistent and realistic visual content. Imagen 3 is able to realise companies' creative visions with high fidelity by creating images that match given text scripts with unparalleled accuracy.
- Chirp 3: Chirp 3, Google's model for voice production and voice understanding, has been updated with new features. Instant Custom Voice, one of the most striking innovations, makes it possible to create artificial but very realistic customised voices from a voice sample of only 10 seconds. In this way, companies will be able to personalise call centre voices with a voice unique to their brand or create special voice assistants with a small amount of data. In addition, Chirp 3's advanced transcription capability can recognise and transcribe each speaker individually when transcribing multi-speaker recordings. This provides remarkable accuracy and ease of use in use cases such as meeting summarisation, podcast solutions or call recording analysis.
- Lyria: Introduced as the first corporate artificial intelligence model that produces music from text, Lyria can convert simple written commands into 30-second high-quality music clips. The model, which can produce rich compositions that can capture subtle nuances in different genres from jazz to classical music, enables companies to quickly create the original music they need. For example, creating royalty-free tracks with the appropriate atmosphere for marketing campaigns, product launches or in-game music becomes possible with Lyria. With Lyria, brands can effortlessly produce signature soundtracks that fit their image, thus increasing the emotional impact and brand consistency of their content.
- Veo 2: Veo 2, the new version of Veo, Google's text-to-video production model, is now more than just a video creation tool, but a full-fledged video editing platform. With the new features announced, developers will be able to remove unwanted background objects or logos from videos created with Veo 2 (inpainting), expand the frame of an existing video to fit different screen sizes (outpainting), and add smooth transitions between two frames (interpolation). There's also the ability to control cinematic camera angles and shooting techniques, adding a professional touch to auto-generated videos. These developments make Veo 2 more than just a model that creates short clips, but a solution that supports the end-to-end process of creating and editing videos.
Security and Compliance
- Google Unified Security (GUS): Introduced a new unified security platform that brings together features such as threat intelligence, security operations, and secure browsing.
- Sovereign AI Services: Google offers sovereign cloud solutions with local partners to meet data sovereignty and regulatory requirements.
Vertex AI Platform Innovations
- Vertex AI Dashboards: New dashboards added to the Vertex AI platform make it easier to monitor the usage and performance of your AI models. With these dashboards, you can track metrics such as the number of requests, latency and error rates in real time; you can detect and fix potential errors faster. Improved visibility and control for developers and operations teams increases the reliability of AI projects.
- Model Customization and Fine Tuning: Google Cloud announced new training and tuning capabilities that let you customize core AI models with your own data sets. Now, Google models such as Gemini, Imagen, Veo as well as open source models can be fine-tuned with company data. This is done in a secure environment, protecting the confidentiality of your data. As a result, organizations will be able to more easily create and manage high-performance models specific to their own areas or use cases in the cloud.
- Vertex AI Model Optimizer: This new feature uses Google's infrastructure and model knowledge to produce the most appropriate response to a given query in the most efficient way. Model Optimiser automatically selects and guides the ideal model for each request, taking into account the quality, speed and cost preferences specified by the developer. For example, if your application prioritises low cost in some operations and the highest accuracy in others, Model Optimizer provides the best balance by activating the appropriate Gemini model and related tools if necessary. In this way, the burden of selecting separate models for different scenarios is eliminated and the optimum user experience is delivered seamlessly.
- Live API: Enabling real-time, multi-modal interactions, Live API supports sending streaming audio and video directly to Gemini models. With this feature, AI agents can understand not only text, but also live speech and video and react instantly. For example, when you integrate an agent with the Live API, it can listen to an ongoing meeting and provide a real-time summary, or instantly analyse a security camera feed. For developers, the API makes it significantly easier to create human-like conversational experiences such as voice interfaces, virtual assistants or interactive broadcast applications.
- Vertex AI Global Endpoint: Google Cloud introduced the Vertex AI Global Endpoint to ensure consistent AI response times on a global scale. With this global endpoint, requests to the Gemini model are intelligently routed across multiple regions to balance capacity. Even in times of heavy traffic or service outages in a particular region, requests are automatically transferred to available infrastructure in another region. For the end user, this means that their applications receive fast and reliable AI responses regardless of geographic location. Especially for companies serving a global user base, it becomes possible to provide scalable AI service to the whole world from a single API endpoint.
Agent Ecosystem Innovations
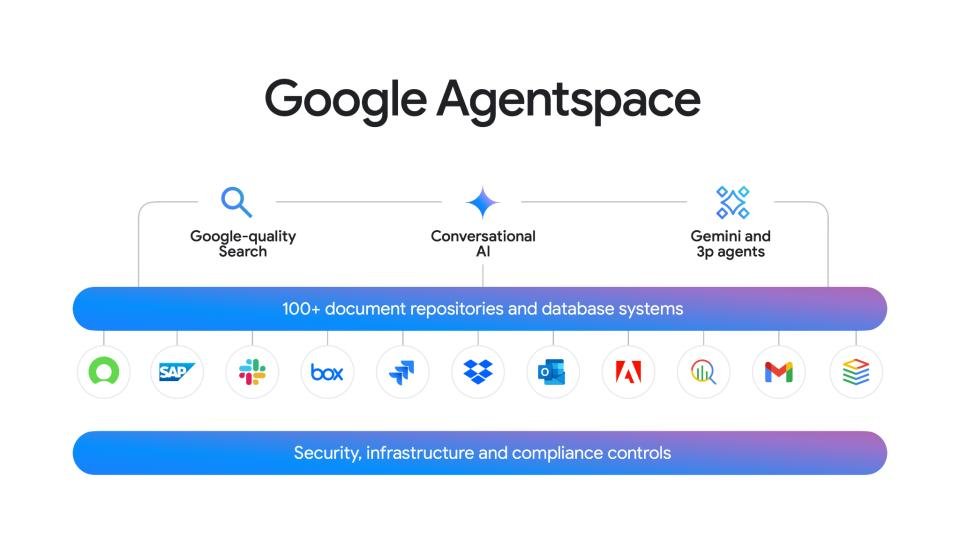
- Agent Development Kit (ADK): Introduced at Google Cloud Next '25, ADK provides developers with an open source framework that makes it easy to build and manage multiple AI agents. ADK simplifies building systems where multiple specialized agents work together in a full-stack approach. Thanks to its high-level APIs, it is possible to build hierarchies of agents that delegate complex tasks to each other or execute them in parallel with little code. With ADK, for example, you can build an AI agent that automates your workflow with just 100 lines of Python code. In addition to tight integration with Gemini models, the framework offers the flexibility to use models from different vendors such as Anthropic, Meta, Mistral AI via Vertex AI Model Garden. It can also integrate with common tools such as search, code execution and popular libraries such as LangChain to provide agents with various capabilities.
- Agent2Agent (A2A) Protocol: Google announced Agent2Agent (A2A), an industry first that enables different AI agents to communicate with each other. Developed as an open standard, the A2A protocol enables agents from different manufacturers or platforms to communicate securely and work together. Thanks to this standard, which was shaped with the contribution of more than 50 business partners (such as Salesforce, SAP, ServiceNow), it is aimed to create a common language between different AI solutions of a company. For example, an AI agent used in customer service and an inventory management agent will be able to communicate and coordinate directly over the A2A protocol. This common protocol is an important step that will increase the return on corporate AI investments by facilitating the integration of parts in multi-agent ecosystems.
- Agent Garden: Agent Garden is a library of ready-made agents bundled with ADK that speeds up the work of developers. Sample agents, connection templates and workflow components provided by Google and the community are readily available in Agent Garden. This library makes it possible to adapt an existing template for common use cases instead of writing an agent from scratch. Agent Garden also includes over 100 predefined connectors, so you can integrate your agents into Google Cloud data services such as BigQuery, AlloyDB or enterprise applications such as Salesforce, SAP, etc. with minimal effort. As a result, Agent Garden gives developers a quick start, reducing the development time of multi-agent projects.
- Agent Engine: Introduced by Google Cloud, Agent Engine is a managed runtime environment designed to run agent-based applications at enterprise scale. Integrated with ADK (but also supporting standalone frameworks), Agent Engine makes it easy to manage the lifecycle of agents from concept to production. This platform automatically handles the context information, memory management, scaling needs, security and access control that multiple agents use, all in the background. Agent Engine, for example, allows you to specify what historical information an agent that has been active for a long time will remember or how long it will keep it in memory. And with real-time monitoring and evaluation features, you can examine the performance and behavior of agents in production and make improvements. In short, Agent Engine provides an enterprise-class operating environment for agents, enabling developers to focus on agent logic without getting bogged down in infrastructure details.
- Grounding with Google Maps: Google announced Google Maps integration for grounding AI agents with geographic and positional data. Vertex AI agents will now be able to use Google Maps' fresh and reliable location data when providing their answers. This means agents can provide more accurate and up-to-date information, especially for location-based questions or tasks. For example, a delivery scheduling agent will be able to provide the most accurate addresses, directions or distance calculations instantly when integrated with map data. Grounding with Google Maps strengthens agents' connection to the world, ensuring that their outputs are consistent with reality and reducing the risk of inaccurate spatial information.
- Agent Gallery: Offered as part of the Google Agentspace product, Agent Gallery acts as a gallery that lists all the AI agents available within the company in one place. Through this gallery, employees can easily discover and access the available agents in the company's AI inventory. The gallery shows a mix of Google's off-the-shelf agents (e.g. NotebookLM), custom agents created by in-house development teams, or third-party agents integrated from partners. This centralized view makes it easy for employees to find the right agent for the task they need and start using it immediately. All in all, Agent Gallery is a step forward in promoting the widespread adoption of AI across the organization and ensuring that every employee can benefit from these agents.
- Agent Designer: Agent Designer, one of the Agentspace innovations, was introduced as a software development tool that enables even users with no coding knowledge to create their own artificial intelligence agents. Offering a visual interface with drag-and-drop logic, Agent Designer divides the work the user wants to do into steps and automatically creates the agent flow that will perform these steps. For example, if a human resources specialist wants to design an agent to summarize and rate job applications, they can define this process on Agent Designer and create their own agent in a few clicks. With its no-code approach, this tool takes artificial intelligence development from the monopoly of technical teams to a level where all departments can innovate.
- Idea Generation agent: One of the sample agents introduced by Google, “Idea Generation” is an artificial intelligence agent developed to support internal innovation and brainstorming processes. This agent autonomously generates many ideas, puts them through a tournament-style evaluation process and highlights the strongest ideas according to the criteria set. For example, a product development team can define criteria (such as cost, impact, feasibility) for the agent to find new feature ideas, and the agent can generate a large number of suggestions and report the best few suggestions based on these criteria. The Idea Generation agent supports employees' creative thinking and decision-making processes, helping innovative ideas to emerge and accelerating innovation by highlighting alternatives that might otherwise be overlooked.
Infrastructure and Performance Improvements
- Ironwood TPUs: Google introduced Ironwood, the seventh generation of TPU (Tensor Processing Unit) chips that form the heart of the cloud infrastructure running AI models. Ironwood TPUs deliver 10 times the performance of previous generations of TPUs, providing a giant leap forward in Google's AI supercomputing architecture. Each Ironwood TPU “pod” contains more than 9,000 chips, totaling a massive 42.5 exaflops of computing power. This capacity is specifically designed to meet the scale needed to train and operate massive models such as Gemini 2.5. Through improved performance and energy efficiency, Ironwood significantly increases AI output per unit cost.
- Google Distributed Cloud (GDC): Some organizations cannot keep their data in the public cloud due to regulatory requirements or data privacy reasons, but they also need AI solutions. Google offers a solution to this problem by moving cloud infrastructure to customer data centers with Google Distributed Cloud. With the announcement made at Next '25, Google's most advanced AI models, the Gemini series, will be available in on-premises environments through GDC. Together with hardware partners like NVIDIA and Dell, this solution enables companies to leverage Google's AI capabilities on their premises or within specific country borders. In short, GDC aims to bring modern AI everywhere without compromising data sovereignty as a platform that brings the innovative power of the cloud to the customer.
- vLLM Support in TPU: The vLLM library, developed in the open source world for distributed and efficient running of AI models, has gained popularity especially in PyTorch-based large language model applications. At Next '25, Google announced that vLLM is now supported on Cloud TPU infrastructure. This means that users with a vLLM-optimized model on GPUs will be able to run the same model on TPUs without drastically changing their code. As TPUs can typically deliver high-performance and cost-effective AI operations, this support aims to make AI workloads faster and more affordable. In particular, researchers and companies that have adopted the PyTorch ecosystem gain the flexibility to leverage Google Cloud's TPU performance while maintaining existing workflows.

Application Development and Management
- Application Design Center: Designing and deploying modern applications in the cloud can be complex and error-prone with traditional methods. Google Cloud's Application Design Center is a new service developed to simplify this process. Available as Public Preview, the center allows platform administrators and developers to design, edit and configure application architectures on a visual canvas, following best practices. For example, when you want to create a microservice-based web application, you can drag-and-drop tools to bring services, databases and other components together and draw the relationships between them. Application Design Center allows you to view these templates directly as code (Infrastructure as Code) and work on them simultaneously with your teammates when needed. By deploying the ready application template with a single click, it enables the automatic creation and configuration of all background cloud resources (VM, container, database, etc.). As a result, this service shortens the time from application design to deployment and helps you ensure that critical issues such as security and scalability are properly addressed.
- Cloud Hub: For organizations that run a large number of applications and services in the cloud, the ability to manage all of these pieces in one place is essential. Cloud Hub is Google Cloud's newly announced service that acts as a centralized dashboard for your entire application ecosystem. Cloud Hub visualizes your applications and infrastructure resources spread across different projects and provides a holistic view of their status, performance and cost. For example, if an application has multiple microservices, databases and queuing systems, Cloud Hub associates them under a single application and shows their overall health and connectivity. It also provides centralized information on operational issues such as usage quotas, resource reservations, maintenance events and support requests. This enables both developers and operations teams to take critical management actions for their applications from a single interface, and quickly identify and resolve issues. Cloud Hub is currently available to all users in the preview phase.
- App Hub: Another part of Google Cloud's application-centric approach, App Hub is a modeling and inventory system that connects distributed application components. It allows you to create an application map by identifying your applications along with the services and workloads they contain. In this way, instead of seeing individual VMs, containers or functions in traditional cloud consoles, you can see all related services and their status together in the App Hub interface, for example under the name “Order Processing Application”. With the update made with Next '25, App Hub has been extended to integrate with more than 20 Google Cloud products such as GKE (Google Kubernetes Engine), Cloud Run, Cloud SQL, AlloyDB. With this integration, App Hub provides a live view of your applications' infrastructure, making it easier to understand which components are in what state and how they interact with each other. This application-centric view makes it easy for administrators to visualize dependencies and assess the impact of changes, especially in microservice architectures.
- Application Monitoring: Google Cloud announced a new feature called Application Monitoring to make monitoring and debugging tools more application-centric. This feature enriches the existing Cloud Monitoring system with application context. Available in Public Preview, Application Monitoring automatically tags collected log, metric and tracing data with the application and service context to which it belongs. This means that when you examine an error log, for example, instead of just seeing the timestamp and virtual server, you can directly see which component of the application the log came from. And with application-specific dashboards and metrics views, you can track the performance of each application individually. For example, defining a custom latency alarm for an e-commerce application's purchasing service is made easy with Application Monitoring. This innovation enables DevOps and SRE teams to diagnose problems faster and stay focused even in complex cloud environments by monitoring in context.
Developer Tools and Database Innovations
- Gemini Code Assist Tools: Google has introduced Gemini Code Assist, an AI-powered code assistant to its ecosystem to increase the productivity of software developers. Gemini Code Assist, which can be integrated as an add-on to popular development environments such as Android Studio, VS Code, JetBrains IDEs, offers artificial intelligence support at every step of the code writing process. For example, it performs functions such as code completion suggestions, detecting and correcting errors, annotating existing code, or automatically generating a block of code to perform a specific task. As part of Next '25, it was announced that new agent-based capabilities were added to Code Assist tools. In this context, specialized artificial intelligence agents are offered for certain tasks in the development lifecycle. For example, the Application Prototyping agent helps to create a prototype of a working application (with both its interface and backend) by defining requests only in natural language. Similarly, the Application Testing agent can take care of automated test cases and bug catching for the code you write. All these tools aim to enable developers to automate routine and time-consuming tasks and focus on more creative and critical work. Google announced that these new Code Assist agents are now available to the developer community as previews.
- Firebase Studio: Another innovation that aims to radically improve the developer experience is Firebase Studio, which was added to the Firebase platform. Firebase Studio was introduced as a cloud-based and agentic development environment; in other words, we can say that it is an IDE that is supported by the Gemini model in the background and delegates some of the operations to autonomous agents. This environment is designed to manage the entire process of modern applications from concept to go-live in one place. Firebase Studio includes a variety of tools and templates to help developers quickly build full-stack applications. For example, the App Prototyping agent allows you to sketch your app using only natural language commands. You describe what kind of app you want (e.g. “a recipe sharing app”) and the agent creates the appropriate database schema, API endpoints, interface sketch and even AI flows. You can immediately deploy the resulting prototype to Firebase App Hosting, test it on a real URL and get feedback. Firebase Studio comes with more than 60 ready-made app templates and integrates with all the infrastructure services Firebase already offers. Developers can import their existing code projects and continue editing them in the cloud or create custom templates for their teams. In short, Firebase Studio, as a fully browser-based IDE, offers an innovative platform that accelerates and simplifies application development with AI.
- AlloyDB AI: One of the most remarkable innovations announced in the field of database management was AlloyDB AI. AlloyDB, Google's high-performance PostgreSQL compatible database service, is now equipped with built-in artificial intelligence capabilities. With AlloyDB AI, developers will be able to run AI workloads directly within the database and perform vector derivation and similarity search for text and visual data. For example, it becomes possible to create vector embeddings from the description texts in a product catalog table and search for the closest similar product on these vectors. Normally, such semantic searches require a separate vector database and AI model, but AlloyDB AI handles this within the database with familiar SQL commands. Google has integrated the ScaNN infrastructure, which it also uses in its own internal services, into AlloyDB, enabling very fast indexing and querying of vector data. In addition, AlloyDB AI allows you to run new types of queries by combining the output of AI models (for example, a text summary or category tag) with traditional table data. This will allow developers to continue using their existing databases without having to think of the data layer as a separate system when building generative AI applications. AlloyDB AI is considered as a step that smartens databases in the era of Gen AI.
- MCP Toolbox for Databases: An important announcement at the intersection of databases and AI agents at Google Cloud Next '25 was MCP Toolbox for Databases. MCP is a protocol known by the abbreviation “Model Context (or Control) Protocol” and is also included in ADK. MCP Toolbox refers to the toolset that enables AI agents to connect directly and securely to enterprise databases using this protocol. Traditionally, in order for an LLM-based agent to write to or retrieve information from a database, custom coding, API integrations and security control mechanisms had to be built in between. MCP Toolbox greatly simplifies this process by providing ready-made connectors and query transformation modules for popular databases (e.g. AlloyDB, BigQuery, PostgreSQL, etc.). For example, with MCP Toolbox, a customer support agent can translate a natural language question from an end-user into a SQL query that fits the company's database and get the answer, or vice versa, add a transaction record to the database - all within predefined security rules. The toolkit also integrates corporate requirements such as authentication, authorization and auditing during data access. As a result, MCP Toolbox for Databases bridges the gap between AI and existing enterprise data infrastructures, making it possible to quickly implement data-driven AI applications.
İlginizi Çekebilecek Diğer İçeriklerimiz
Her iki model türü de doğal dil işleme (NLP), bilgisayarla görme ve diğer alanlarda güçlü uygulamalara olanak tanır. Ancak, bu modellerin yapılarına, uygulamalarına ve avantajlarına baktığımızda belirgin farklar var.
At the Google Cloud Next '25, many innovations were announced in the field of artificial intelligence and cloud that will closely interest developers and decision-makers. In this article, we compile and summarize the main topics introduced at the event.