As someone who has been working on both the corporate and consulting side of the industry for many years, I can say that the biggest challenges and threats for companies is to correctly describe the need for transformation, prioritize the areas of need and development throughout the organization and implement the roadmaps created in parallel with them at the management level.
It is no longer inevitable that companies focus on Digital Transformation, Data and Analytics strategies and act using next-generation technologies and techniques so that they do not lose their competitive advantage. At times, however, we also observe that digitalization or analytical transformation is only viewed from the perspective of technology investment or the use of ready-made solutions.
In order for data and analytics transformation programs to be sustainable and effective, the ultimate goal must be to change the perspectives of the company's most important employees and decision makers on data and analytics issues, increase data literacy across the organization, and implement infrastructures where they can use data in every tactical and strategic decision.
As Komtaş Ecosystem companies that have been providing data and analytical solutions to leading institutions in their sectors for nearly 30 years, we have developed a methodology that we call dNA Strategy, taking these needs into account. We have found the opportunity to apply this consulting service, developed by Analythinx, Damalink and Digitallency with dozens of local project experiences and differentiates according to sectors, in many companies of different scales and sectors, from our expertise centers in projects and ecosystem companies with global technology partners.
Using the dNA Strategy framework model, we are able to analyze the data and analytical competencies of organizations in the framework of nearly 40 dimensions in the 9 main headings shown below.

The presence of industry-specific analytical scenario pools within the framework enables business units and next-generation technologies such as Big Data, IoT, Cloud and advanced analytics, ML, AI techniques to talk and evaluate business opportunities that can be realized using new generation technologies such as Big Data, IoT, Cloud and advanced analytics, ML, AI techniques, and at the same time increase awareness of these issues.
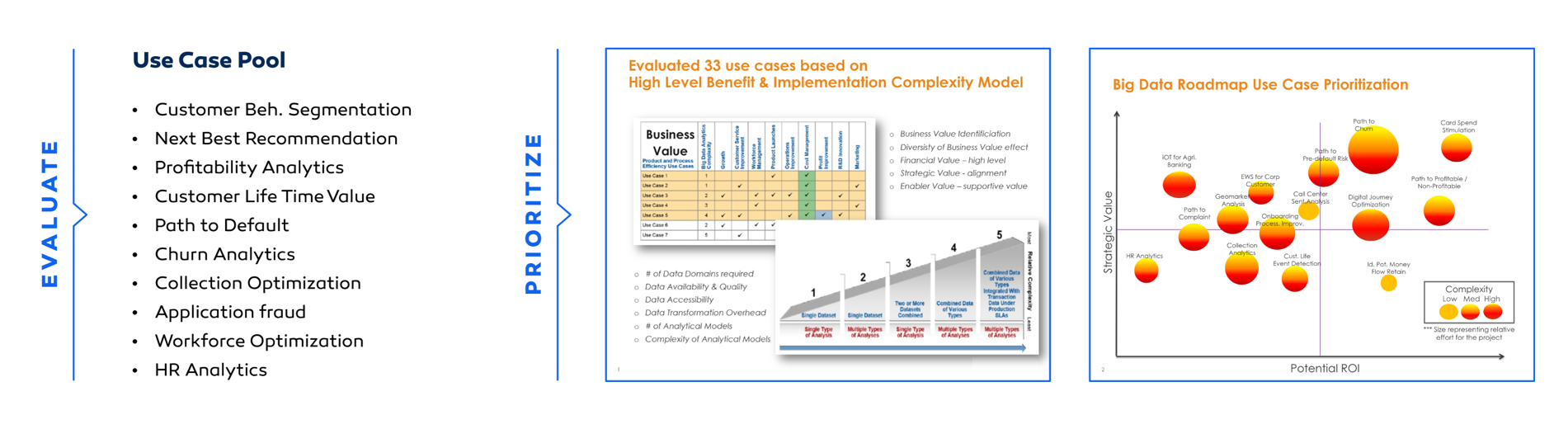
In order to effectively implement these prioritized scenarios and minimize operational risks, we also question competencies such as Data Governance (Data Quality, Data Catalog, Data Security, Governance Processes) and Data Consolidation (Data Warehouse/Business Intelligence), and we are able to reveal all the needs in the big picture.

I can say that the biggest difference of Dna study compared to similar approaches is in the level of presentation of the results of the work. When you do this type of work with the most senior employees of a team with field experience, who have done projects on related topics, you have the opportunity to ask the right questions based on the “lessons learned” in the projects, more than just imagining the project. You can feed into the results of strategy work by asking the problems and questions that you may encounter at the beginning of the development phase.
From this point of view, you can see not only exciting analytics or business intelligence projects, but also structural transformation projects and investments such as Data Lake, Data Warehouse, IoT architecture, and human resource capability/capacity development areas to operate these systems and processes, and organizational business model changes. When we turn the results of the interviews into a manageable and traceable program output from a holistic perspective, the organization can continue its transformation journey with our own resources if it wants to implement these initiatives with its own resources or at certain points with solution providers like us.
Finally, I would like to list some of the key success factors and criteria that will make a difference, regardless of who or with which model you are doing it for companies considering this type of transformation;
- The program is sponsored by a C-level administrator, providing in-house support
- An experienced team with field experience on the consulting firm side to carry out the work
- Scenario prioritization with the participation of all business units and information technology units
- Incorporating prototype studies into the process (such as data quality work on a sample dataset, experience migrating to the cloud with a rapid acquisition model, or demo/prototyping some ready-made analytical scenarios)
- Develop an activation roadmap supporting centers of excellence and hybrid work models at points such as analytics and data governance within the organization
- There has certainly been a break in the transformation roadmap and the inclusion of rapid acquisition projects
In recent years, we have had many clients from different sectors where we have implemented this program, although the needs, outputs and roadmaps of each of them are specific to that institution, the common thing we saw in all was the transformation projects that immediately follow the work.
To give a few examples, after our work in a large healthcare group in just 3 weeks last year, we identified ERP transformation, digitalization initiatives, data/analytics program proposals and technology investment projects together and helped start the transformation.
As a result of our work with a joint Dna Strategy program for 5 companies belonging to a large holding in the past year, we created customized transformation programs and target architectures according to each subsidiary's own needs and development areas. Currently, the transformation programs in these companies continue intensively together with advanced analytical projects on cloud technologies, primarily data management.
There have been examples that we have done in the banking and insurance sector in much earlier years, although these institutions that started investing in data and analytics much earlier seem advantageous in this respect, they have to undergo a much more challenging transformation due to the difficulty of changing their current architecture and processes. In this regard, in our studies in these institutions, we also sought answers to questions such as how to create migration models with minimal effort and risk, how to meet needs with hybrid structures and data/technology migration models. In the two different banks we have conducted similar studies, technology and human resources were transformed in line with the analytical priority maps that emerged after the strategy study, and many successful advanced analytical projects were signed.
If you also want to make a difference with Data and Analytics transformation to increase your company's competitiveness, you can always reach out to me or info@komtas.com to evaluate how the Dna Strategy work will contribute to this process.
Özgür Kaynar
Analythinx - Managing Partner
İlginizi Çekebilecek Diğer İçeriklerimiz
This guide has provided you with both conceptual insights and practical code examples to help you get started with container technology on Google Cloud. Explore, experiment, and take full advantage of the modern development paradigms that containers and GKE have to offer. Happy coding!
"Amazon'un boyutu" ifadesini aradığınızı düşünün. Arama uygulaması şirketi mi yoksa nehri mi kastettiğinizi nasıl bilecek? Başka bir deyişle, yapay zeka belirli bir görevin bağlamını nasıl anlayabilir?