Data occupies a very important place in our lives today, so much so that in 2020 alone, people tweeted about 500,000 tweets each day, sent 306.4 billion emails, and generated an average of 1.7 MB of data per second. As a result, by the end of 2020, about 44 Zettabytes of data made up the entire digital universe. Looking at the economic scale of the business, 70% of the world's GDP has gone digital, and by 2025, 200 Zettabytes of data will be stored on cloud systems. In short, the entire digital universe formed by 2020 will grow exactly 5 times at the end of a 5-year period with incredible growth!!
It is very difficult to imagine the magnitude of the data we will have, the economic value it will provide and the know-how it will contain. In this case, we involuntarily question how we can make use of such a volume of data, what values we can generate at every opportunity. It's not hard to predict that everyone will respond to AI, no matter what industry, but even in an Earth where data is generated at such a rapid rate, and in changing conditions, it can already lead to difficulties in improving and maintaining continuity, no matter how successful the models it produces. This emerging consistency problem also brings new operational workloads in AI and ML. However, the concept that has entered our lives as mLOps in recent years can help organizations close a significant AI skills gap; 68% of managers describe their organization's AI skills gap as moderate to extreme, while 27% rate it as significant or extreme, and according to the assessment, mLOps and AI processes are expected to be the strongest aspect of solving the problem.
In this respect, MLOps considers a whole range of applications and tools that bring automated self-development, versioning, control and automation to the processes of training, testing, deploying, monitoring and managing AI solutions. This automation allows teams to discover new ideas, reduce development and operational costs, quickly receive feedback on them, and focus on the most efficient points in AI processes.
Artificial intelligence projects involve a lot of research and experimentation because the problems solved by AI are often variable, not linear. To approach the problem systematically and efficiently, AI model implementation needs to be fast, reliable, and reproducible by automating testing, training, and deployment pipelines. In addition, the project team needs to standardize infrastructure from development to production and carry out solid safety planning from start to finish.
It is an important element of MLOPS to verify that the AI model continues to work as expected and to ensure continuity. Since machine learning algorithms are adapted to improve without human guidance in response to new data and experiences, monitoring is vital. End-to-end management and automation functionality of processes are vital to detect model drift over time and develop new models that are consistent against it.
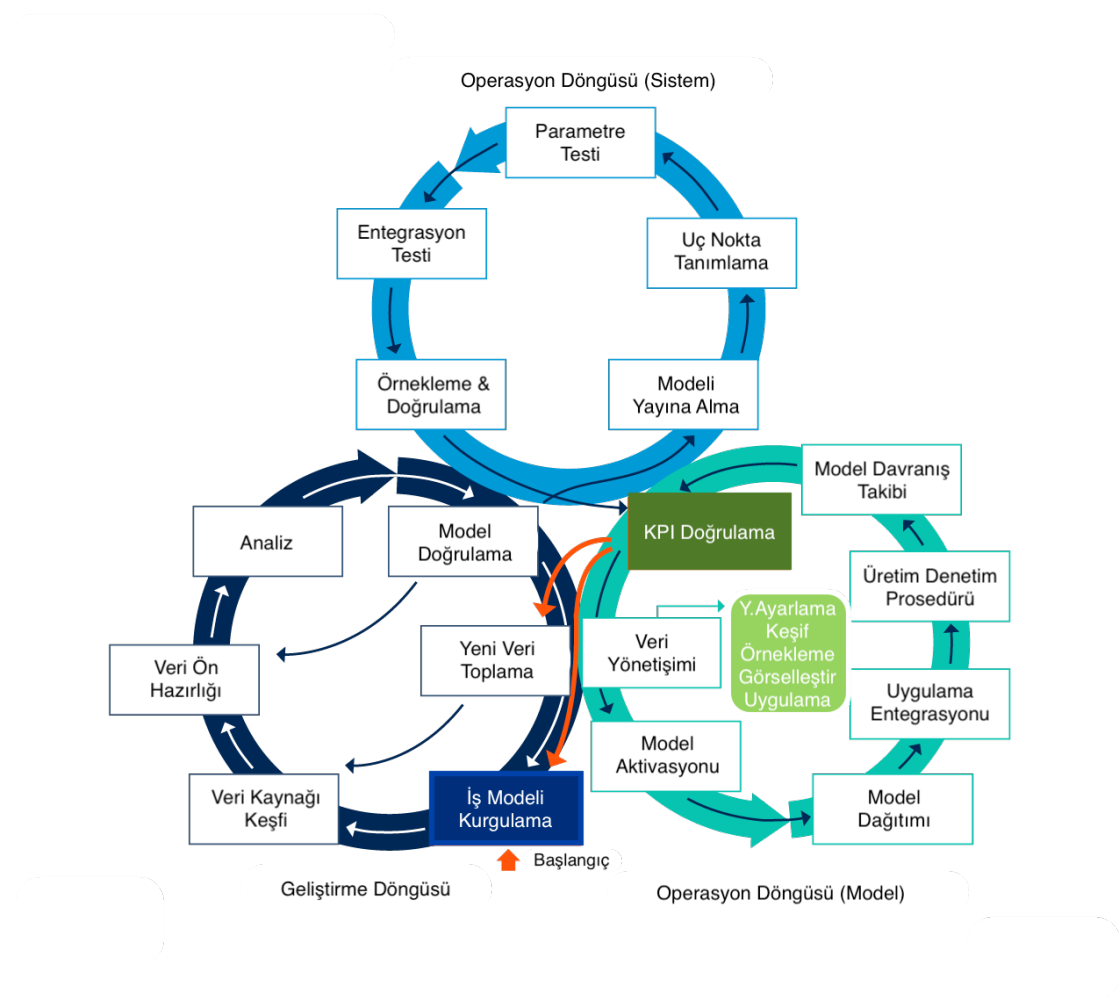
What Does MLOPs Benefit Institutions?
MLOps drives results by focusing on the entire lifecycle of the design, implementation and management of Machine Learning models as a solution to these problems in AI applications. The operational process is covered in 3 sub-headings, Model Development, Model Operation, and System Operation, as you will see in the mLOps Lifecycle graph above.
- mLOps aims to mature the core principles of DevOps in AI applications; automation (as opposed to software development over and over again); positioning (as opposed to one-time use); process (integration, testing and release); and end-to-end scripting with infrastructure issues, applying the same process each time with new data and updating It focuses on model building, dynamic reporting, and keeping continuity under control in this way.
- Successful and mature MLOps systems require a more diverse, well-equipped, large-scale team of data scientists, data engineers, software engineers, R&D specialists, process engineers, and business development officials of all levels. Instead of repeatedly producing core lines of work that include iterative machine learning models within MLOps and many experiments in development phases, this team creates a system that can self-improve and manage all these operational processes end-to-end by automating all these operational processes.
- In addition to the standard unit and integration test that we are used to seeing in DevOps processes, the machine learning test must validate and retrain AI models, at which point MLOps automatically takes responsibility for this process on itself.
- When models go into production, many things can change, especially the accuracy rate and model performance. With data profiles, end-user preferences will evolve and influence subsequent processes, current state of critical assumptions and parameters are incorporated into the system with healthy execution of the mLOps process.
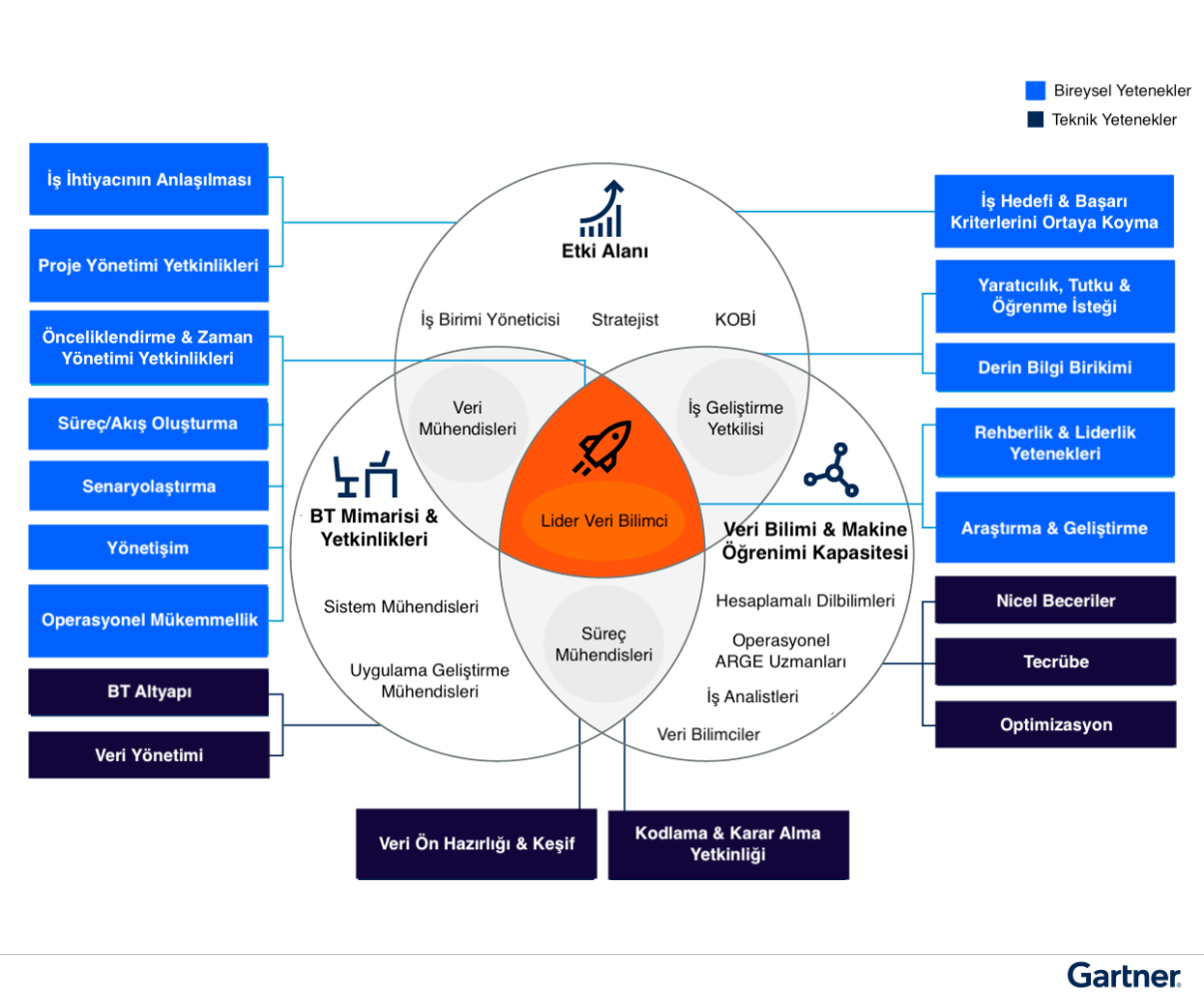
Roadmap to the MLOps Process
The path to MLOPS and more effective ML development and deployment depends on selecting the right people, processes, technologies, and operating models with a clear connection to business issues and outcomes. At this point, Gartner puts the competency and role-specific operating model of an ideal MLOps process on a broad perspective as above.
- People with different roles and responsibilities should meet in common; companies should invest in pre-structured solutions while also investing in bringing AI practitioners and data scientists together in a single application. Business and field experts can create use cases with business models, data science professionals can drive innovation in machine learning models, and data and machine learning engineers can use automated machine learning tools to bring together rapid machine learning models.
- It is necessary to incorporate automation into processes; mLOps aims to create versions of models with reusable software, automated data preparation and collaboration. Thus, a data scientist can reuse or accelerate use cases based on one-off generated models.
- Acceptable, realistic success metrics and criteria must be established; It is critical to express success criteria, make a preliminary preparation and integrate performance standards.
MLOps is at the heart of next-generation AI applications!
As artificial intelligence and machine learning are being adopted across the enterprise, model structures must be explainable; the underlying data must be reliable when building models; their effects can be measured; results sustainable; system designs must be scalable and self-educate and mature models in new versions by making assumptions from erroneous estimates.
Machine learning is like all the other powerful tools that technology brings. When used correctly, it can help build data-driven decision-making processes. On the other hand, improper distribution harms the intended business results. One of the great advantages of machine learning is the speed of analysis and insight on a large scale, but if misguided, models can result in inadequate or even poor decisions at the same speed and scale. To avoid this, during the design phase of our MLOps architectures, we need to determine all our AI and machine learning processes in a controlled and standards-compliant manner, perform product and acceptance tests in real life, and set success criteria at levels that will please the end user.

As Komtaş, we continue to build end-to-end mLOps processes of organizations together with our Dataiku technology, which was a Leader in the Gartner Magic Quadrant List in 2020 & 2021.
İlginizi Çekebilecek Diğer İçeriklerimiz
Veri analisti (Data Analyst), verileri toplayan, analiz eden ve bu verilerden anlamlı içgörüler çıkararak işletmelere stratejik kararlar almalarında yardımcı olan bir profesyoneldir.
Makine Öğrenimi Mühendisi (Machine Learning Engineer), veri analizi ve yapay zeka algoritmalarıyla çalışan, makinelerin öğrenmesini ve veri odaklı kararlar almasını sağlayan sistemleri geliştiren bir profesyoneldir. Bu mühendisler, istatistik, programlama ve veri bilimi becerilerini kullanarak, iş süreçlerini otomatikleştiren ve optimize eden çözümler oluşturur.